DeepSeek-R1 Clearly Outperformed OpenAI's o1 pro mode In My Ant Sim Test
I tested DeepSeek-R1 against OpenAI's o1 pro mode by having both program an ant simulation from the same prompt. DeepSeek-R1 generated a far superior simulation. As AI models become increasingly commoditized, I think this shows again that AI is a hardware revolution, not a software revolution.
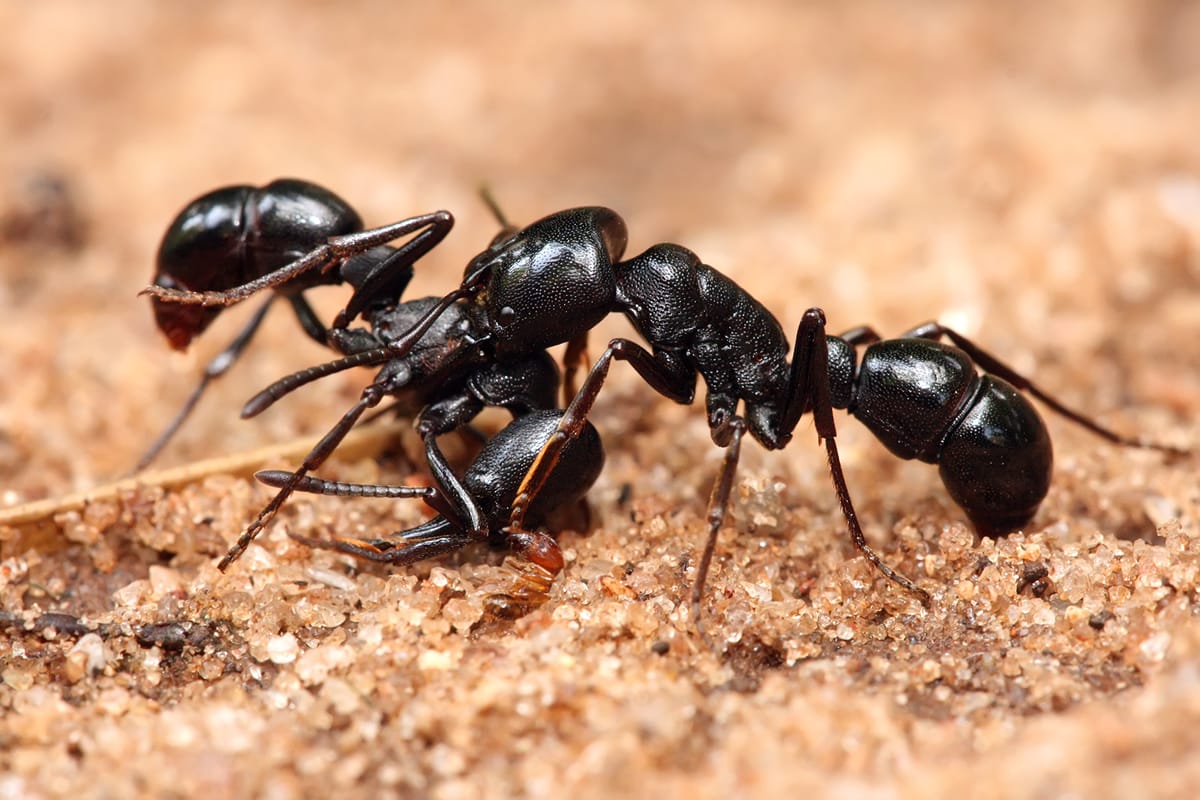
This is the author's opinion only, not financial advice, and is for entertainment purposes only. The author holds a beneficial long position in NVIDIA Corporation. The author receives no compensation for writing this article and has no business relationship with OpenAI, Inc. or DeepSeek.
In December, I compared OpenAI's o1 pro mode with the other versions of ChatGPT by prompting them to simulate an ant colony. Before that, in September, I used my ant simulation test to evaluate the programming capabilities of o1-preview. I like this test because writing such a simulation is certainly not a standard task and requires some transfer. Besides, you don't need to understand programming to judge the result.
In recent days, DeepSeek-R1, the latest open-source reasoning AI model from Chinese AI startup DeepSeek, has created quite a buzz. To be honest, I was very skeptical that a Chinese startup with a reported budget of $5.6 million could develop an AI on par with OpenAI's models. So I decided to compare DeepSeek-R1 with OpenAI's o1 pro mode using my ant sim test.
I gave both models exactly the same prompt, which was entered only once, with no subsequent corrections or suggestions from me. To ensure a fair game, I also cleared the memory of my ChatGPT account beforehand (I did not have a DeepSeek account before, this was my first prompt here). I took the prompt from my ant sim test in December without changing it:
Create a simulation of an ant colony foraging for sugar using separate HTML, CSS, and JavaScript files. The ant nest should be positioned in the center of the screen, with 25 sugar piles and 100 ants distributed randomly. Each sugar pile should contain a discrete number of sugar units, approximately normally distributed with a median of around 10 units, and its visual size (radius) should reflect the quantity of sugar it holds. The ants should move in a random, frequently changing manner. If an ant reaches the edge of the screen, it should simply bounce back by changing direction. When an ant encounters sugar, it should pick up one unit, carry it directly to the nest, and then return to roaming randomly. While carrying sugar, the ant should emit a scent trail that gradually fades over time, and this trail should be displayed in gray. Other ants that detect this scent trail should follow it back to the sugar source. After an ant delivers sugar to the nest, it should temporarily ignore scent trails to prevent ants from gathering near the nest where trails converge. Each time a sugar unit is removed from a pile, the pile should visibly shrink to reflect the reduced amount of sugar remaining. Take care to use distinct, appealing visual representations for the ants, the nest, and the sugar piles, and ensure that ants never become stuck and that the simulation runs smoothly.
DeepSeek-R1 took almost three times as long to think about as o1 pro mode, but the superiority of its ant simulation is so clear that I almost don't need to describe it (see below): DeepSeek-R1's simulation meets my requirements exactly, while o1 pro mode's simulation is, to put it mildly, in need of some improvement (surprisingly, it seems to be even worse than the simulation o1 pro mode generated for me in December):
Ant simulation by DeepSeek-R1 (generated January on 27, 2025)
DeepSeek-R1's ant sim is a perfect realization of my prompt.
Ant simulation by OpenAI's o1 pro mode (generated on January 27, 2025)
In the simulation generated by o1 pro mode, the scent trails are invisible and the ants are stuck to them.
This proves it to me once again: NVIDIA's GPUs are the engine of this industrial revolution
DeepSeek-R1 demonstrates that the AI revolution is essentially a hardware revolution, not a software revolution, as AI models become increasingly commoditized. It's speculated that DeepSeek only has about 50,000 of NVIDIA's H100 GPUs. But we simply don't know how many of NVIDIA's GPUs bypassed export restrictions and ended up in China and are available to DeepSeek. We also do not know how much power the servers behind DeepSeek-R1 are really consuming and how much money DeepSeek is really burning. We can all check the performance of DeepSeek-R1, but not the effort behind it.
However, using the incredible success of DeepSeek as a bearish argument for NVIDIA is, in my opinion, a misunderstanding of this development. Even if only fifty thousand H100 GPUs were used to train DeepSeek-R1, what will the developers behind this triumph be able to do with NVDIA's next Blackwell generation of GPUs if they have access to them? We are still at the beginning of this industrial revolution, and many different great AI models from different developers will disrupt all parts of our economies. I think they will have one thing in common: they will all run on NVIDIA GPUs.
Follow me on X for frequent updates (@chaotropy).