Choosing Google's TPUs Over NVIDIA's GPUs Is Like Choosing A Zeppellin Over A Jumbo Jet
Are NVIDIA's AI chips still "so good that even when the competitor’s chips are free, it’s not cheap enough", as Jensen Huang said in March? Short answer: In my opinion, yes, they are still "so good".
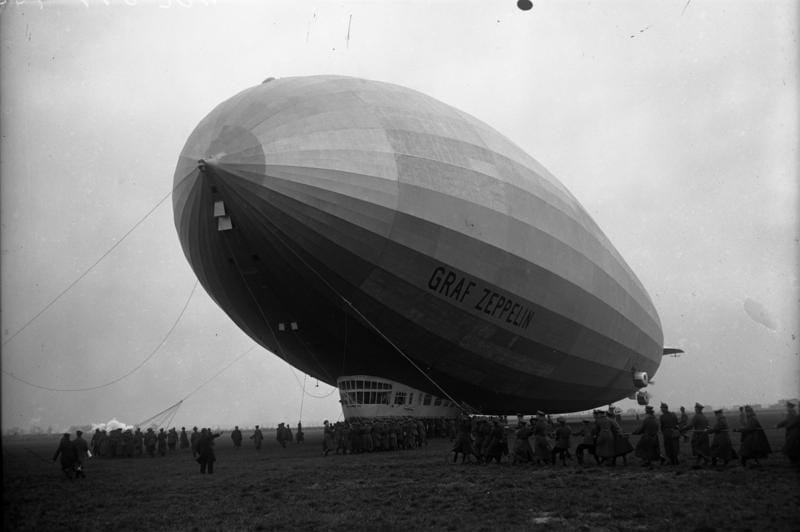
This is the author’s opinion, not financial advice, and is intended for entertainment purposes only. The author holds a beneficial long position in NVIDIA Corporation. All calculations and conclusions in this article are based on estimates and may not be accurate. The author receives no compensation for writing this article and has no business relationship with any of the companies mentioned.
On Monday, July 29, Apple Machine Learning Research published the scientific background of its “Apple Foundation Model” (AFP). According to the paper, this model (AFP-server) was trained on 8,192 of Google’s TPU v4 chips, and apparently no NVIDIA GPUs were used for training (although this was not explicitly mentioned). Jensen Huang said in March that NVIDIA’s AI chips are “so good that even when the competitor’s chips are free, it’s not cheap enough.” Is that still true if Apple uses Google’s TPUs to train its AI instead of NVIDIA’s GPUs? Let’s crunch the numbers.
In the following, I have chosen FLOPS as the metric to compare the chips. Using a different metric may lead to different results. FLOPS (floating point operations per second) is a measure of the computing power of computer chips and correlates to the performance of matrix operations such as those required for AI training. We are in the teraFLOPS (10¹²) to petaFLOPS (10¹⁵) and exaFLOPS (10¹⁸) range for the chips discussed.
According to the Google Cloud website (as of August 1, 2024), a TPU v4-Pod (4,096 x TPU v4) costs $32,200 per hour on-demand. Thus, the computing capacity to train the AFM-server cited in the paper costs $64,400 per hour on-demand. 8,192 TPU v4 have a combined processing power of up to 2.25 exaFLOPS (8,192 x 275 teraFLOPS). In comparison, Google’s newer TPU v5 can compute up to 459 TeraFLOPS, and a cluster of 8,960 TPU v5 costs $42,000 (TPU v5p) and can compute up to 4.11 exaFLOPS.


One of NVIDIA’s H100 SXM5 ( up to 3,958 teraFLOPS) costs between $3.15 and $4.49 per hour on-demand at NEBIUS AI, depending on the reservation time and the volume of GPUs used (as of August 1, 2024). In our example, it would make sense to choose the largest volume (up to 128 GPU chips), which would cost between $3.15 and $3.52 per GPU per hour. Let’s assume that we chose the shortest reserve time and therefore the most expensive offering in this category. So a cluster of 128 GPUs would cost about $450 per hour (128 x $3.52) and can compute up to 507 petaFLOPS (128 x 3,958 teraFLOPS). So for $64,400 (actually for $63,980) per hour, we could rent 142 full clusters of 128 H100 SXM5 with a total compute capacity of up to 72 exaFLOPS (142 x 507 petaFLOPS).
According to this calculation, we would get the 2.25 exaFLOPS of computing power used by Apple Machine Learning Research for $2,012 per hour by using clusters of NVIDIA’s H100 SXM5, making the use of NVIDIA’s GPU about 32 times cheaper than using clusters of Google’s TPU v4.
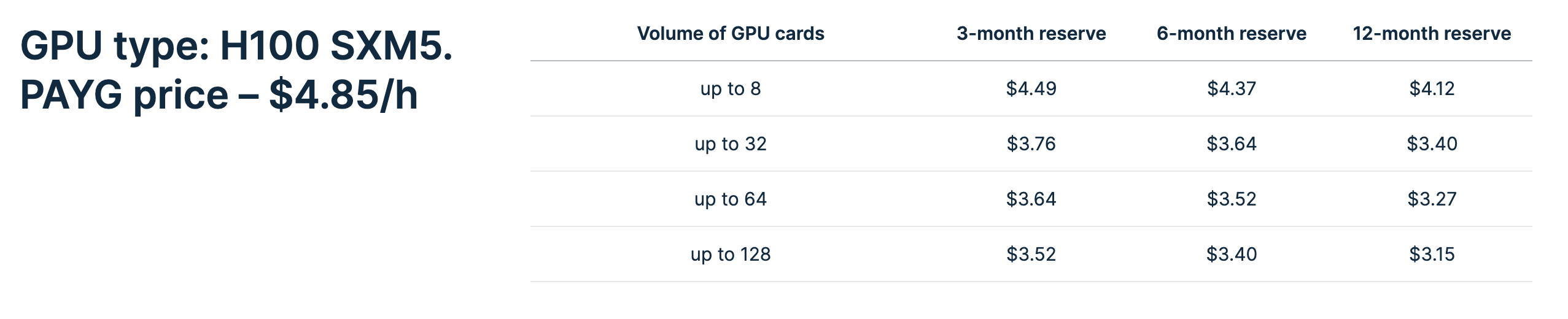
Now that we’ve talked about Hopper (H100), let’s take a look at the upcoming Blackwell generation. A Blackwell GPU is expected to cost between $30,000 and $40,000, the H100 chips currently cost about the same today, and a single Blackwell core is expected to compute up to 20 petaFLOPS. NVIDIA plans to market 2 Blackwell GPUs as a GB200 superchip with a corresponding computing power of up to 40 petaFLOPS, with an estimated price of $60,000 to $70,000.
To the best of my knowledge, there is no on-demand pricing information available for the GB200. If we assume that on-demand use of a GB200 costs between $12 and $15 per hour (after all, the chip is more power efficient than the H100), then we would get between 4,293 and 5,366 GB200s for $64,400 per hour, giving us a computing capacity between 171.7 and 214.6 exaFLOPS. So we would get the 2.25 exaFLOPS of computing power used by Apple Machine Learning Research for $677 to $844 per hour. This would be between 76 and 95 times cheaper than using Google’s TPU v4-Pod as mentioned in the paper.
For comparison, an airship like the Hindenburg, built in 1936, could carry about 70 passengers from Frankfurt to New York in about 64 hours. Depending on the type, a jumbo jet (Boeing 747) can carry about 420 passengers the same distance in 8 hours. From this point of view, a jumbo jet is about 48 times more efficient than the Hindenburg. So the difference in efficiency between NVIDIA's upcoming Blackwell GPU and Google's TPU is even greater than the difference between a modern jet and a 1930s Zeppelin. Who would choose the Zeppelin?
Of course, this whole argument is based on a single metric, and comparing GPUs to TPUs is a bit like comparing apples to oranges, but it does show how cost-effective NVIDIA’s chips really are. In addition to the pure processing power discussed above, there are many other reasons to prefer NVIDIA’s GPUs, which are much more versatile than TPUs. The power of CUDA to optimize AI on NVIDIA’s GPUs has also been ignored in this discussion. But back to the original question: yes, NVIDIA’s chips are still “so good” in my opinion.
Follow me on X for frequent updates (@chaotropy).